Background: Scoping
“the Evidence Act establishes a framework to expand agency capacity and enable access to the federal government’s confidential data for evidence building (that is, provide value) while protecting those data.”(1)
Goals
- Understand the Evidence Lifecycle and how it applies to the class
- Learn about the project use case
- Know the rationale and expectations for group work
The evidence life-cycle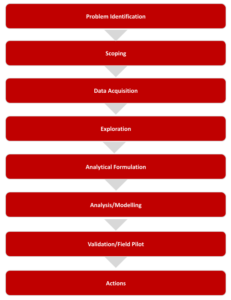
Our goal is to provide an overview of how to work with data to create evidence – how to go from project to product to practice. The process is often called scoping, design thinking, developing a theory of change, or identifying the “so that” reason that you’re doing the project at all. It’s likely that everyone in the class is familiar with the process, but the class will unpack each part of the process in a systematic way. Step 1 is that senior management or state legislators identify a problem and want analysts to provide data and evidence upon which to base decisions (I). Then the project begins.
The project use case
The project use case is the Multi State PostSecondary Report (MSPSR) which was developed from a training class just like this one. The high level “ask” of KYStats was to help institutions in Kentucky determine the performance of their programs, particularly to respond to legislation like the College Transparency Act and the Kentucky Right to Know Act.(3). Collaboration between the Kentucky Center for Statistics (KYSTATS) and the Kentucky Council on Postsecondary Education (CPE) led to a relatively strong understanding of employment and wage outcomes for a specific subgroup: recent postsecondary degree earners who find in-state UI-covered employment. The MSPSR was developed by a group of Kentucky, Ohio, and Indiana government analysts (4) who went on to use Kentucky and Indiana data to understand the labor market outcomes of individuals who acquire Kentucky postsecondary degrees and credentials. The MSPSR can be used to answer questions for institutions like what are the employment rates for graduates from my institution, or higher level questions like what are the 1, 2 and 3 year earnings and employment outcomes for education graduates? READ MORE
Work in teams
Data Science is a team sport. The class is structured in both lecture format and in teams so that participants can work hands on with the data and learn from each other. The teams are designed so that each team member brings a different skill set to the team project: one team member might have expertise in policy, another in statistics, another in coding, and another in data. READ MORE
Exercise
Readings
- Introduction to Advisory Committee on Data for Evidence Building: Year 2 Report (pp 11-14)
- Supplemental Materials for Advisory Committee on Data for Evidence building (p13-18)
References
- Advisory Committee on Data for Evidence Building. Advisory Committee on Data for Evidence Building: Year 2 Report. Washington DC. 2022.
- Kit T. Rodolfa ADU, Matt Gee, Rayid Ghani. An Experience-Centered Approach to Training Effective Data Scientists. Big Data. 2019;7(4):249-61.
- Cunningham J, Hui A, Lane J, Putnam G. A Value-Driven Approach to Building Data Infrastructures: The example of the MidWest Collaborative. Harvard Data Science Review. 2021.
- Kuehn D. Better Data for Better Policy: The Coleridge Initiative in Ohio. https://www.urban.org/sites/default/files/2022-05/Better%20Data%20for%20Better%20Policy%20-%20the%20Coleridge%20Initiative%20in%20Ohio.pdf